Problem
Around a year ago, an apparel and accessories brand came to Cotera looking for a new, strong recommendation model to replace its current one.
The Head of Retention knew they should be generating higher incremental revenue from moving customers across different products.
Yet they found themselves stalled leveraging their ESPs’ recommendation engine, as it was unable to adjust for the nuances of their business. And any attempts at testing stand-alone recommendation engines lacked the turnkey integration with their ESP.
They wanted a product recommendation model that could automatically:
- Strategically select products based on customers’ willingness to pay / price point
- Cross-sell to higher margin verticals and products
- Only recommend products in stock
- Integrate with their ESP to send and update recommendations with names and images in real-time
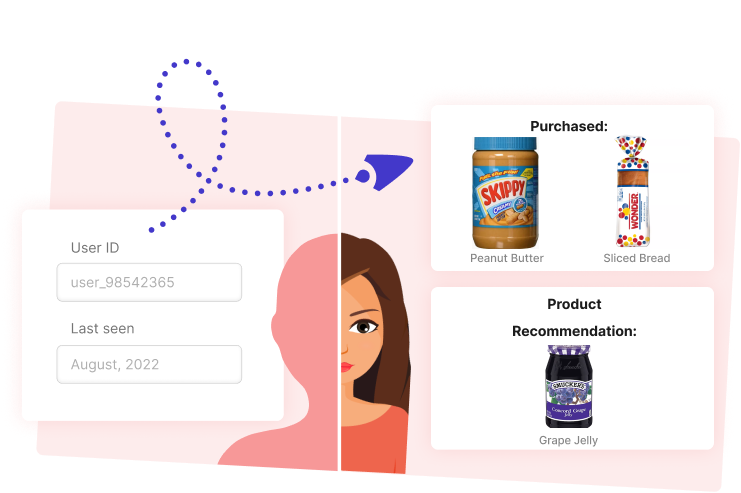
Impact of Cotera
- 30% increase in AOV
- 4x more likely to purchase the product shown
- Cotera paid for itself from the incremental revenue generated off ONE email campaign
Prior to Cotera, the brand faced several problems:
1) Automated recommendations through their ESP frequently featured loss leader and low price point items
Many existing ESP recommendation engines aren’t built for recognizing deeper patterns that reveal the exact needs, desires, or WTP (willingness to pay) for a customer.
Because of this, recommendations being made were ineffective toward getting more value out of customers. The apparel brand found that the most common products recommended were all low margin and low price point, which did no good to customer AOV at all.
2) Items recommended through their ESP were out of stock or seasonal
Since recommendations wouldn’t even always end up being available to customers, many complaints arose of bad user experiences. And this ended up losing the company a great deal of potential incremental revenue.
3) Prior stand-alone product recommendation engines had bad integrations with their ESP
Other recommendation engines that this brand tried weren’t reliable either. There were consistent cases of missing images or incorrect product links when transferring the recommendations to their ESP, which was obstructive for brand image and led to extensive amounts of missed revenue.
So because they didn’t want to risk inconsistency and inaccuracy, they turned to Cotera.
Solution
This apparel brand leveraged Cotera’s product recommendation model which could now take into account:
- A customer’s price sensitivity (based on their purchase values)
- Similar customers’ purchases
- Product category
- Stock/inventory of the product
- Browsing/Abandoned Cart Behavior
- Age of Product (New Products vs Existing Products)

How it Works
1) Data is constantly collected, updated, and used to generate quality recommendations
We don’t just decide on recommendations using one customer’s own data — we collect and use data from the ENTIRE database of customers to generate more accurate assumptions about what similar customers might enjoy. AND we can identify underlying trends to make connections across categories of products, like finding the relationship between a black leather jacket and a pair of brown rubber boots.
2) Recommendations are seamlessly uploaded into the ESP and automatically ready for use
Our model always double checks any product recommendations to ensure everything is in-stock, and that any images, links, and names are all correctly uploaded into the brand’s email and marketing platforms.
Impact
Through Cotera’s recommendation model…
- Customers who were shown Cotera’s recommendations had on average 4x the likelihood of purchasing compared to customers who were shown items recommended through their ESP.
- Customers who were shown Cotera’s recommendations on average had a 30% higher AOV than customers who purchased through either the ESP’s recommendations or who were shown “New” or “Trending” Items.
- Companies were able to pay for the entire cost of Cotera through the incremental revenue generated by sending, on average, just one marketing email with Cotera’s recommendations.
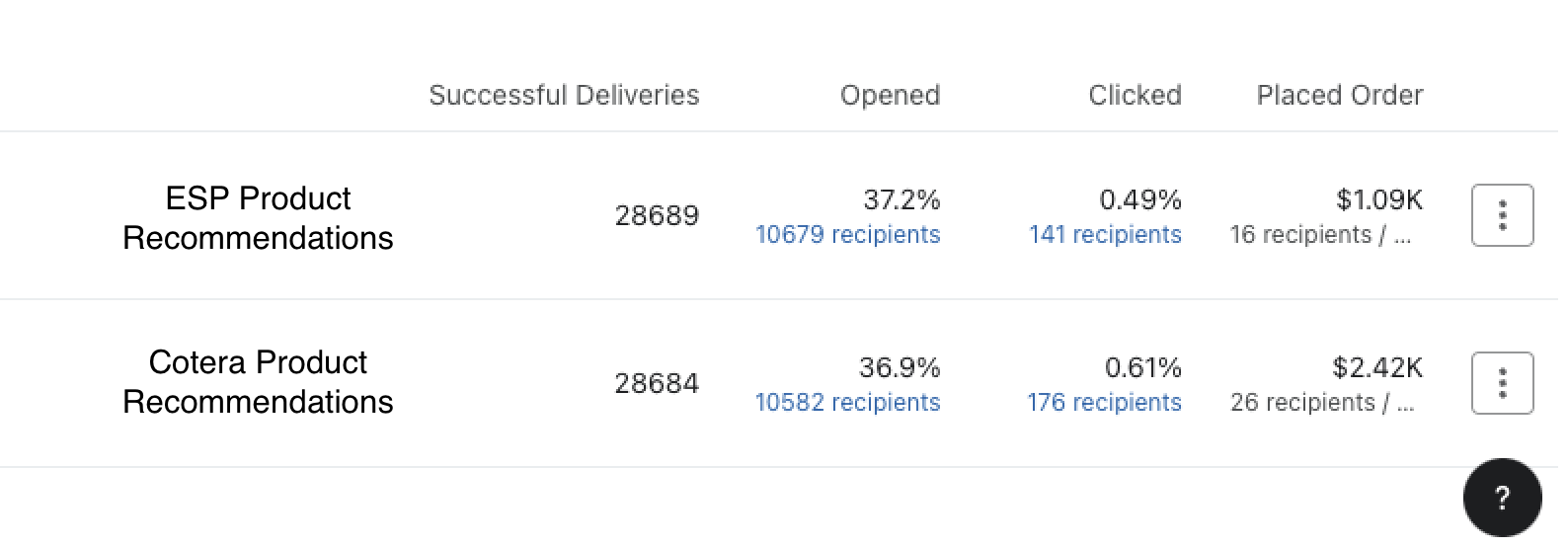
Interested in stepping up your recommendation engine game? Book a demo here!